Would you talk to a chatbot therapist? Are you comfortable with an artificial intelligence tool that helps screen for heart disease? What about one that suggests an unusual — but potentially life-saving — treatment?
Whether your instinct is to balk at — or cheer — the growing use of AI in medical care and research, one thing is certain: Changes are coming, and it’s a good idea to start thinking about how we may want the law to address them, says I. Glenn Cohen ’03, the James A. Attwood and Leslie Williams Professor of Law at Harvard Law School.
Cohen recently co-authored a paper in the medical journal JAMA about the ethical and legal concerns raised by one such tool — ambient listening apps — that help providers record and take notes during patient visits. But Cohen, who is also the faculty director of Harvard Law’s Petrie-Flom Center for Health Law Policy, Biotechnology & Bioethics, says that AI is being integrated into health care in many other ways, too, including research, medical imaging and analysis, and even diagnosis and treatment.
Because many AI models can parse enormous quantities of data and even learn as they go, these technologies have the potential to transform care for millions of people, Cohen says. But they also raise a multitude of questions. How much control do — or should — people have over their health information? Must providers inform patients when they use AI in their treatment — even if it’s just to document what happened during an appointment? And should those whose data is used to train AI derive some benefit from their contribution?
In an interview with Harvard Law Today, Cohen describes some of the surprising ways AI is transforming health care today and how we can use the law to balance innovation with the need for safe, reliable, and equitable medicine.
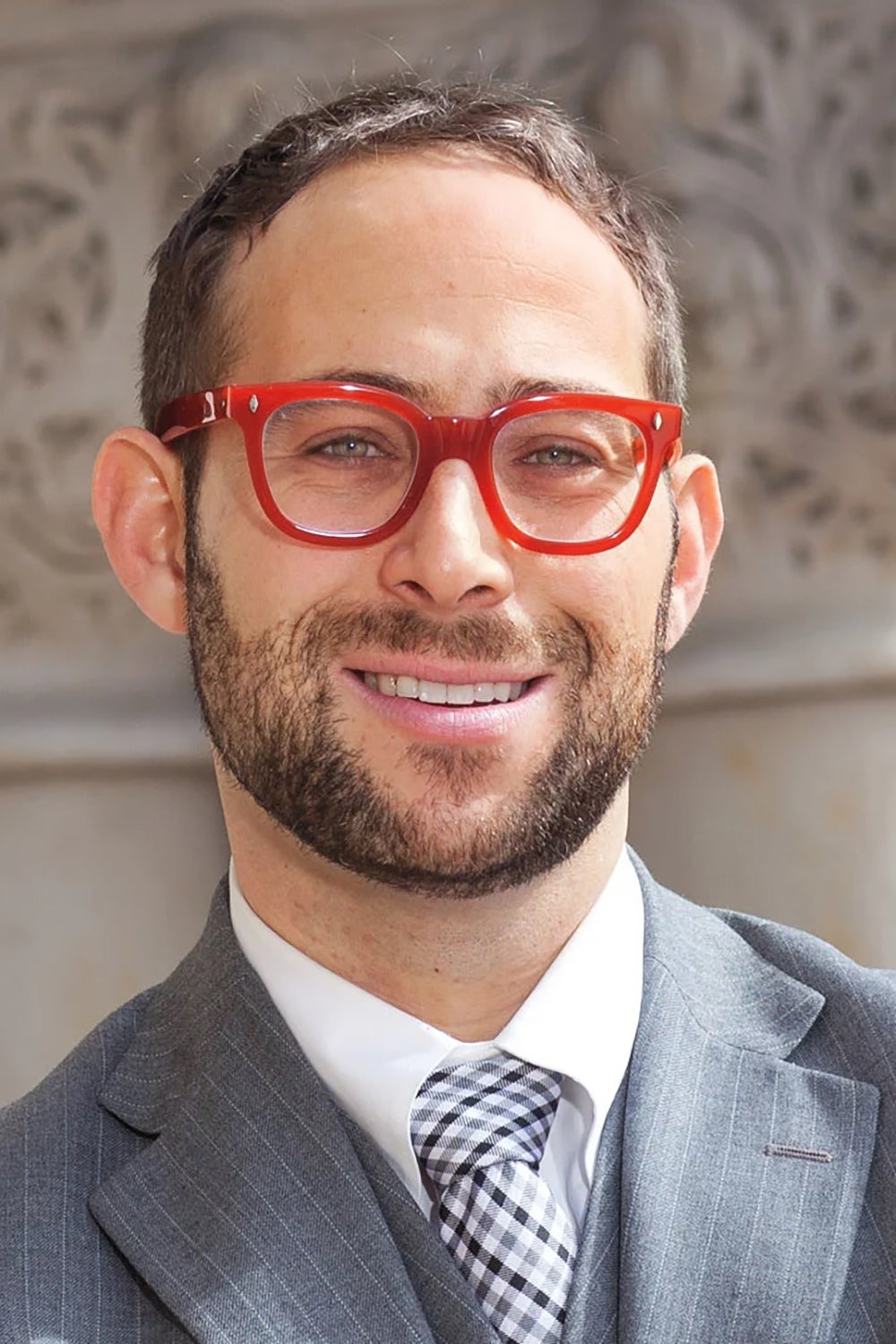
Harvard Law Today: Let’s start with the basics. How is AI being used in health care and medicine right now?
I. Glenn Cohen: I’ll give you a few examples, but I’ll note that we’re seeing a wide range of ways in which it’s being used. Let’s say someone gets a colonoscopy and there’s a lesion. We can use AI to help determine whether that lesion is malignant or benign. Or take someone who is trying to get pregnant through in vitro fertilization. There is a lot of variation in how much of what are called follicle-stimulating hormones to give, and AI can help determine a starting amount. It can also help determine which embryos to implant that might have the best possibility of a successful pregnancy.
There are also mental health chatbots, some of which are designed for the mental health space, others of which are more general — large language models, for example. Then there is the Stanford Advanced Care Planning Algorithm, which is sometimes called the “death algorithm,” because it’s used in Stanford hospitals to try to predict three- to 12-month all-cause mortality of patients. The idea is that a certain score would trigger a conversation with the physician about end-of-life planning. There’s also something called ID XDR, which was developed for diabetic retinopathy, and it can be used relatively autonomously for a screening decision.
Another common thing we’re seeing right now is what’s called ambient listening or scribing. The idea is that, rather than having your doctor type and look at you while you’re meeting with them, a device records the conversation and then summarizes it using AI. Then, the physician is supposed to review it before it gets finalized and goes into your chart.
HLT: What are the major ethical considerations that we need to — or will need to — confront related to the use of AI in medicine?
Cohen: I think about this in terms of steps related to the build process. The first concerns are about patient governance and patient rights over their data. For example, where does the data come from? What kind of consent is needed? How much identification can or do we need to do? What is the risk for privacy of the data? How representative is the data set? I’m a white guy, in my 40s, living in Boston. I’m dead center for most of the data that’s used to train on machine learning in medicine. But that’s not true for everybody, especially if we broaden to outside the U.S. into low- and middle-income countries.
Then once you build a model, there are the questions about how we know that it’s ready for prime time to use with real patients. Who does the validating, and which regulator or combination of regulators might look at it? How do we deal with regulatory structures from different countries? How much transparency can you have with the data? How do you deal with questions about trade secrecy and intellectual property?
And once you’re ready to use the model with real world patients, what do these patients have to be told? What kind of consent is necessary? How do we know whether deployment in a new context is raising additional implications or variations of the quality of the model? How much should the AI be learning as it goes versus it’s static on something like a “factory setting” — in other words, adaptive versus locked. And then again, to the extent that there are complaints about discrimination or bias, what forms of regulation or litigation are relevant there?
And lastly, there are questions about what I would call “broad dissemination.” You’ve got models that are successful and working. How do we ensure that all patients whose data contributed to the model actually receive a benefit from it? How do you ensure that we can scale up to really democratize expertise?
HLT: Earlier, you mentioned ambient listening tools, which are designed to aid medical providers during care sessions. You recently wrote an article for JAMA about the legal and ethical issues implicated by these models. What are your biggest concerns?
Cohen: Well, first, I want to be clear that we’re comparing these tools to the baseline, which is physicians trying to ask questions and record answers while seeing a patient — that’s not great. There are a lot of distractions. Doctors can’t make eye contact. It’s exhausting. And even human scribes make errors. So, I don’t want to present this as though it’s only AI that introduces problems.
That said, what kinds of problems do we see with these tools? One is transcription errors — a doctor says “.5 milligrams,” but what’s captured is five milligrams. That’s a big difference in terms of a drug and the effect it might have on a patient. There are also hallucinations, where the tools have generated text that was not part of the conversation. Another issue is that ambient scribing devices are typically not regulated by the FDA. Instead, private companies are the ones making key decisions. We also know from other forms of human-computer interaction that automation bias is a real problem — meaning that over time, people are less likely to catch errors or to disagree with what was written. So, there is a risk that errors won’t be caught.
Informed consent is important here, too. This is about recording someone — in many jurisdictions, it’s actually legally required to get consent by all participants to a conversation, and if you don’t, there may be criminal or civil penalties. Also, patients may have questions about how their information is stored or who is going to access it or whether their clinician will review it. Will this data be used to train future AI — and how do the patients feel about that? And what is the risk that the patient can be reidentified in all this?
Then there are questions about sensitive content. Not every conversation with a physician involves sensitive content, but if it’s something about drug use, domestic violence, or criminal activity, for example, patients might have specific concerns about these things. You may need to have protocols in place for either deleting or turning off the tool when these topics arise and figuring out how to do that ahead of time.
HLT: As a litigator, you also have some thoughts on how ambient listening tools could impact malpractice lawsuits. What are they?
Cohen: One thing is that these tools could have implications for the number of patient records providers generate. As a provider, you want to avoid what’s called a “shadow record.” With these tools, there is the recording itself, then there’s the AI summary, and there’s the version the physician signs off on. What happens if there are discrepancies between these versions? What happens if you get to the point of discovery in a lawsuit and there are three different records? I think hospital systems should plan to treat the non-signed off versions merely as drafts, and to have a retention and destruction policy set up way in advance of litigation.
HLT: How prepared is our law currently to address some of the challenges you have identified?
Cohen: Some forms of our law — say, malpractice law — are well-prepared. But whether that existing law is going to give the right answer is another question. There have been shockingly few cases involving malpractice relating to artificial intelligence so far. When I talk to malpractice insurers, they also tell me that they’re getting very few claims. We don’t yet know whether that’s because it’s increasingly being adopted, as opposed to having been around for a while.
But there’s a way in which the focus on the standard of care in medical malpractice as the marker of the breach of a duty of care might lead to a certain conservativism. In 2019, we published a paper in JAMA that made this point. Imagine a simple case: you’ve got a standard of care dose for ovarian cancer of a certain chemotherapeutic. In a particular instance, an AI recommends a higher dose for a particular woman. That’s part of the goal of AI: the ability to have more personalization, because it can analyze across many multimodal data sets. The problem is that, at least in the interim period, if you’re a physician, you know that you are liable only if a., an injury occurs, but also b., you didn’t follow the standard of care. That encourages a kind of conservativism. That is, if the AI tells you to do exactly what you were going to do anyway — the standard of care — you think it’s a brilliant AI. But if the AI tells you to do something other than what you were going to do, you start to get nervous, and maybe are going to resist it. That means that the conservativism of the way the standard of care is formulated might create barriers to adoption for physicians.
Now, this is a story that we’ve seen with other new technologies. If you look at MRIs, x-rays, and CT scans, there was a period of time where they were not standard of care either. They were introduced, and there was probably some resistance to them initially. But now, if you fail to order an MRI because you just looked at the patient and said, ‘Well, I was doing this the old-fashioned way,’ you may have breached the standard of care. We may eventually get to a similar place with medical artificial intelligence. But the history of medical tort law suggests that this tends to be a slow process.
HLT: Are there any major gaps in the law right now?
Cohen: Most of the medical artificial intelligence currently being used in the United States does not fall under FDA’s jurisdiction, either because of the ways in which Congress wrote that jurisdiction in the 21st Century Cures Act, or FDA’s own interpretation of how to use its discretion in this space. So, a lot of the stuff, especially things you see built into the electronic health record system, nobody at FDA will ever review. Maybe you’ve got some ex post regulation by tort law or maybe by some other regulator. But much of this is self-regulated, with the possibility of liability on the back end.
There are arguments to say we don’t want to over-regulate. That’s fine, but the line between what gets regulated versus what doesn’t isn’t ideal. It’s based on things like “what’s a device,” or “what’s not a device,” as opposed to “what’s in the highest risk category versus the lowest risk category?” That’s a big gap in how I think this is approached.
HLT: Are there any examples of a technology that previously upended the way medicine was practiced? How did the law adapt to that innovation?
Cohen: Earlier, I mentioned imaging, and I think that’s a good example. Another space where we’ve seen a lot of change is genetic testing. We may have overhyped that to some extent. But there was a period of time where we knew much less about the human genome than we do now. Now, for example, in the fertility sector, genetics is much more important. Genetic counselors as a profession didn’t really exist before that technology.
But with it came new questions. For example, once we developed good genetic testing for heritable diseases, we had a bunch of cases asking the question of whether, if you’re a physician and your patient has a positive test for something that is heritable, but also actively requires some kind of early intervention, and you don’t intervene, is there a risk to that person? And what is your duty to disclose to a family member, if the patient is unwilling to do so? There were analogies of other kinds of duties to warn contexts, including contagious diseases, including in the psychiatric context.
My sense is that over the middle- to long- term, the law, through a combination of self-regulation and some statutory and regulatory law, usually does a pretty good job of accommodating these technologies. But in the short term, there can be a lot of disruption and a lot of questions. And that’s part of what makes my job interesting — I get to worry about them.
Want to stay up to date with Harvard Law Today? Sign up for our weekly newsletter.